Understanding GraphRAG: The Future of Retrieval-Augmented Generation in AI
Table of Contents
Introduction to GraphRAG
In the rapidly evolving field of artificial intelligence, GraphRAG emerges as a groundbreaking approach poised to transform machine understanding and information generation. This innovative technology combines graph-based knowledge representation with advanced retrieval and generation techniques, creating AI systems with enhanced capabilities.
Enter GraphRAG – short for Graph-based Retrieval-Augmented Generation. This innovative approach to AI is designed to bridge the gap between raw data processing and human-like understanding. By combining the structure of graph-based knowledge representation with advanced retrieval mechanisms and sophisticated language generation, GraphRAG is opening up new possibilities in artificial intelligence.
In the following sections, we’ll dive deep into the inner workings of GraphRAG, exploring its key components, its advantages over existing technologies, and its potential applications across various industries. Whether you’re an AI enthusiast, a business leader looking to leverage cutting-edge technology, or simply curious about the future of artificial intelligence, understanding GraphRAG will give you valuable insights into the next wave of AI innovation.
What is GraphRAG?
GraphRAG, or Graph-based Retrieval-Augmented Generation, represents a significant advancement in AI language technology by combining the power of structured knowledge representation with sophisticated information retrieval and context-aware text generation.
At its core, GraphRAG utilizes a graph-based knowledge representation system, where information is organized as interconnected nodes and edges. This structure allows for a rich, multidimensional representation of data, capturing not just individual facts but also the complex relationships between different pieces of information. In this knowledge graph, nodes typically represent entities, concepts, or specific data points, while edges denote the relationships or associations between these nodes. This interconnected structure enables the system to capture nuanced contextual information and facilitates more sophisticated reasoning capabilities.
the above illustration represents a knowledge graph where the “Central Concept” connects to various “Related Entities” through distinct “Relations.” This demonstrates how a primary idea or object is linked to other relevant pieces of information, with each entity having a specific relationship to the central concept.
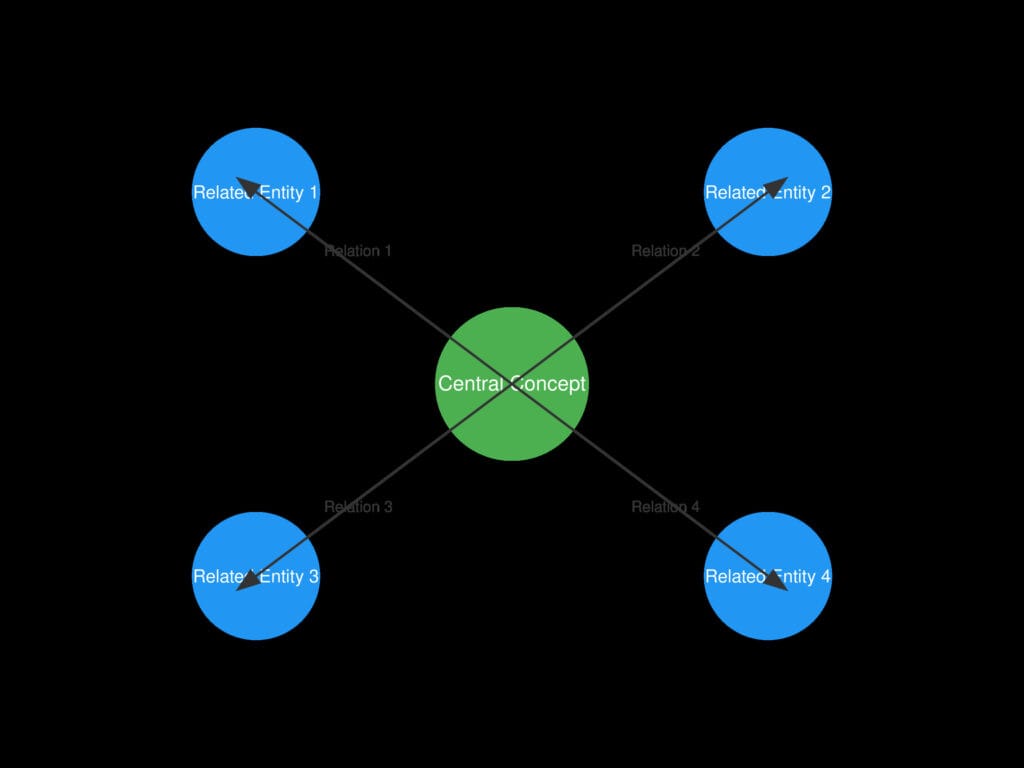
The second key component of GraphRAG is its advanced retrieval mechanism. When presented with a query or task, this system doesn’t simply perform keyword matching but engages in a more nuanced process of semantic understanding. It analyzes the query to grasp its underlying meaning and intent, then uses this understanding to navigate the knowledge graph efficiently. The retrieval process employs advanced graph traversal algorithms that can follow chains of relationships, exploring not just directly related information but also relevant tangential data. This allows for a more comprehensive and context-aware information gathering process. The retrieved information is then scored and ranked based on its relevance to the original query, ensuring that the most pertinent data is prioritized for use in the subsequent generation phase.
In The below illustration, the retrieval mechanism from a knowledge graph is illustrated. It begins with the “Knowledge Graph,” a structured set of interconnected entities. “Query Processing” interprets user inputs, while the “Retrieval Engine” searches the graph for relevant data. The final output is shown as “Retrieved Results,” providing contextually rich information based on the user’s query.
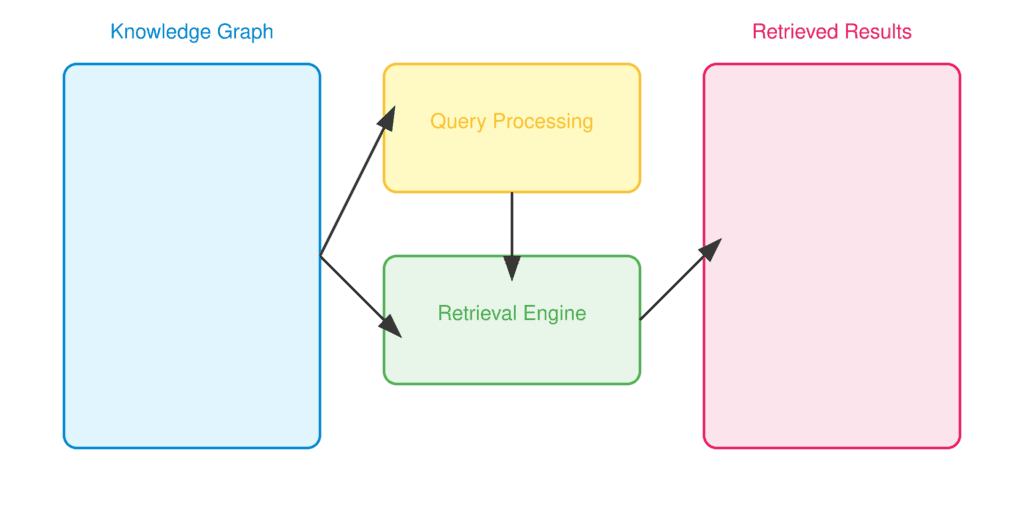
The final component of GraphRAG is its context-aware text generation system. This goes beyond traditional language models by integrating the retrieved information directly into the generation process. The system starts with a powerful pre-trained language model as its foundation, capable of producing fluent and coherent text across a wide range of topics and styles. However, the key innovation lies in how it incorporates the specifically retrieved information from the knowledge graph. This retrieved data serves as a form of dynamic, query-specific context that guides and constrains the language model’s output. The generation process carefully balances between leveraging the language model’s broad capabilities and ensuring that the output remains grounded in and faithful to the retrieved information. This results in responses that are not only linguistically sophisticated but also highly relevant, factual, and contextually appropriate.
The third diagram illustrates how retrieved information is used in text generation. The “Retrieved Information” is processed by a “Language Model,” which integrates additional context through “Context Integration.” The model then produces “Generated Text,” a meaningful response based on the input and context. This process is essential for creating relevant and coherent AI-generated content.
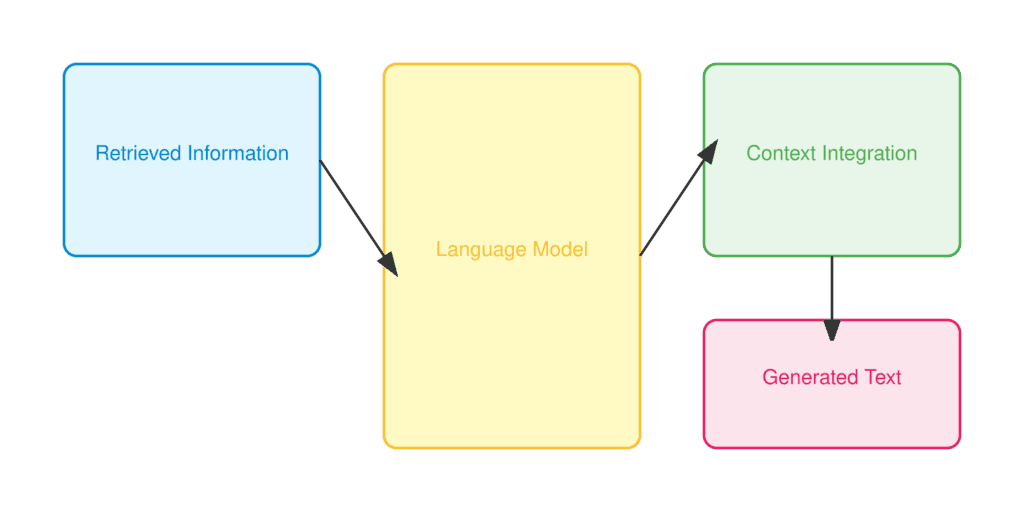
The synergy between these three components – graph-based knowledge representation, advanced retrieval mechanisms, and context-aware text generation – is what gives GraphRAG its power. By leveraging a structured knowledge base, it can access and reason over vast amounts of interconnected information. Its sophisticated retrieval allows it to quickly find and utilize the most relevant pieces of this knowledge for any given query or task. And its context-aware generation ensures that this knowledge is seamlessly integrated into coherent and informative responses. This combination allows GraphRAG to handle complex queries, provide more accurate and contextually relevant information, and even perform reasoning tasks that would be challenging for traditional language models. Whether it’s answering intricate questions, providing detailed explanations, or assisting with complex problem-solving tasks, GraphRAG represents a significant step forward in creating AI systems that can leverage structured knowledge to enhance their language understanding and generation capabilities.
Applications of GraphRAG in Various Industries
The versatility and power of GraphRAG make it a transformative technology across numerous industries and use cases. Let’s explore these applications in detail:
4.1 Natural Language Processing Advancements
GraphRAG significantly enhances various aspects of natural language processing:
- a) Enhanced machine translation: GraphRAG’s graph-based context understanding allows for more nuanced and accurate translations. Traditional machine translation often struggles with context-dependent meanings and idiomatic expressions. GraphRAG, however, can leverage its knowledge graph to understand the broader context of the text being translated. For example, when translating a technical document, it can recognize industry-specific terminology and ensure consistent translation throughout the document. It can also better handle ambiguous words by considering the surrounding context in the knowledge graph, leading to more natural and accurate translations.
- b) More coherent text summarization: Text summarization is greatly improved by GraphRAG’s ability to leverage knowledge graphs. Instead of merely extracting key sentences or generating summaries based on statistical patterns, GraphRAG can produce summaries that capture the essential relationships and maintain overall context. For instance, when summarizing a complex scientific paper, it can identify the key findings, methodologies, and implications, and present them in a coherent manner that reflects their interconnections. This results in summaries that not only condense information but also preserve the logical flow and relationships between different elements of the original text.
- c) Advanced question-answering systems: GraphRAG enables the development of more sophisticated question-answering systems capable of handling complex, contextually nuanced queries. Unlike simple fact-retrieval systems, GraphRAG-powered QA systems can understand the intent behind questions, consider relevant context, and provide comprehensive answers. For example, if asked “What were the long-term effects of the Industrial Revolution?”, the system could draw upon its knowledge graph to discuss economic, social, technological, and environmental impacts, providing a multi-faceted answer that connects various domains of knowledge.
4.2 Knowledge Management Systems
GraphRAG revolutionizes knowledge management in organizations:
- a) Intelligent document retrieval: In large corporate knowledge bases, GraphRAG significantly improves search capabilities. It goes beyond keyword matching to understand the semantic intent of search queries. For instance, if an employee searches for “customer retention strategies,” the system could retrieve not just documents with those exact words, but also related concepts like customer loyalty programs, satisfaction surveys, and churn analysis. This semantic search capability ensures that users can find relevant information more efficiently, even if they don’t know the exact terms used in the documents.
- b) Automated knowledge graph construction: GraphRAG can automatically extract structured information from unstructured text to build and maintain comprehensive knowledge graphs. This is particularly valuable for organizations dealing with vast amounts of textual data. For example, a pharmaceutical company could use GraphRAG to analyze research papers, clinical trial reports, and regulatory documents to automatically construct a knowledge graph of drug interactions, side effects, and efficacy data. This automated process ensures that the knowledge graph remains up-to-date with the latest information, facilitating faster and more informed decision-making.
- c) Cross-domain knowledge integration: One of GraphRAG’s most powerful features is its ability to facilitate the connection of information across different departments or areas of expertise. In a large corporation, for instance, GraphRAG could integrate knowledge from marketing, product development, customer service, and finance to provide a holistic view of the business. This cross-domain integration can reveal insights that might not be apparent when each domain is considered in isolation, leading to more innovative problem-solving and strategic planning.
4.3 Intelligent Chatbots and Virtual Assistants
GraphRAG significantly enhances the capabilities of chatbots and virtual assistants:
- a) More context-aware conversations: GraphRAG enables chatbots to maintain coherence and relevance over extended interactions. By leveraging its knowledge graph, a chatbot can remember context from earlier in the conversation and relate it to new information. For example, in a customer service scenario, if a user mentions a product issue early in the conversation, the chatbot can refer back to this information later when suggesting solutions or offering additional assistance, creating a more natural and efficient interaction.
- b) Improved task completion: The graph-based knowledge representation allows virtual assistants to better understand and execute complex user requests. For instance, if a user asks a virtual assistant to “plan a weekend trip to a nearby city with good museums and restaurants,” the assistant can navigate its knowledge graph to understand the user’s location, find nearby cities, check for highly-rated museums and restaurants, and even consider factors like travel time and accommodation options. This comprehensive understanding of the task leads to more accurate and helpful responses.
- c) Personalized user experiences: GraphRAG can utilize user-specific knowledge graphs to tailor interactions and recommendations. As it interacts with a user over time, it can build a personalized knowledge graph that includes the user’s preferences, past interactions, and relevant context. This allows for highly personalized experiences. For example, a virtual shopping assistant could leverage this personalized knowledge to make product recommendations that not only match the user’s stated preferences but also consider their past purchases, browsing history, and even how these relate to broader trends or similar user profiles.
Comparing GraphRAG to Other AI Technologies
To fully appreciate the capabilities of GraphRAG, it’s helpful to compare it with other AI technologies:
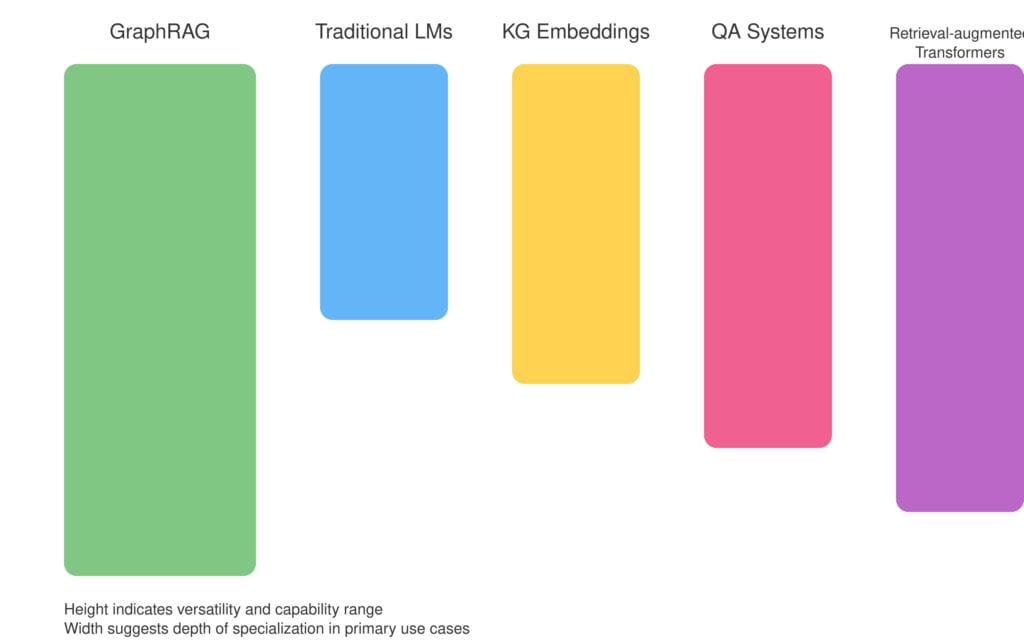
a) Traditional Language Models (e.g., early versions of GPT):
- GraphRAG vs. Traditional LLMs: While traditional language models excel at generating human-like text, they often lack grounding in factual knowledge and can generate plausible-sounding but incorrect information. GraphRAG, with its knowledge graph foundation, can provide more accurate and contextually relevant responses.
- Use case difference: Traditional LMs are suitable for tasks like creative writing or general text completion. GraphRAG is better for tasks requiring factual accuracy and complex reasoning.
b) Knowledge Graph Embeddings:
- GraphRAG vs. KG Embeddings: Knowledge graph embeddings represent entities and relationships in a continuous vector space, which is useful for tasks like link prediction. it goes beyond this by integrating the knowledge graph with advanced language generation capabilities.
- Use case difference: KG embeddings are primarily used for graph-based machine learning tasks. it is more suited for natural language interactions and complex reasoning tasks that require both structured knowledge and language understanding.
c) Question-Answering Systems:
- GraphRAG vs. QA Systems: Traditional QA systems often rely on information retrieval from a fixed corpus. GraphRAG’s graph-based approach allows for more flexible and context-aware answering, capable of connecting information across different sources.
- Use case difference: Basic QA systems are good for factoid questions. it excels in answering complex, multi-step questions that require reasoning across multiple pieces of information.
d) Transformers with Retrieval (e.g., REALM, RAG):
- GraphRAG vs. Retrieval-augmented Transformers: While both enhance language models with external knowledge, GraphRAG’s graph structure allows for more nuanced understanding of relationships between pieces of information.
- Use case difference: Retrieval-augmented transformers improve factual accuracy in generation tasks. GraphRAG is particularly strong in tasks requiring complex reasoning and connection of ideas across domains.
Conclusion
It represents a significant leap forward in AI technology, combining the strengths of structured knowledge representation, advanced information retrieval, and context-aware language generation. Its ability to understand complex relationships, reason across diverse information, and generate nuanced responses opens up new possibilities across various applications.
As research in this field progresses, GraphRAG has the potential to drive transformative changes in how we interact with AI systems, bringing us closer to AI that can truly understand and reason in ways that mirror human cognitive processes. While challenges remain, particularly in areas of ethics and responsible implementation, the future of AI with technologies like GraphRAG is undoubtedly exciting and full of potential.