Diffusion Model: Why you should know about it?
Diffusion models represent a groundbreaking advancement in machine learning, particularly in the realm of generative artificial intelligence. These innovative models have gained significant attention in recent years due to their remarkable ability to create high-quality data samples across various domains, including images, audio, and text. The significance of diffusion models stems from their unique approach to data generation.
Unlike traditional generative models that produce outputs in a single step, diffusion models employ an iterative process that gradually refines random noise into coherent, high-fidelity data. This process, inspired by thermodynamics, involves slowly adding noise to data and then learning to reverse this process, allowing for more nuanced and controlled generation. What sets diffusion models apart is not just the quality of their outputs, but also their versatility and stability. They have shown impressive results in diverse applications, from creating photorealistic images and synthesizing natural-sounding speech to generating coherent text and even assisting in scientific research like molecular design.
The implications of diffusion models extend beyond technical achievements. They are reshaping how we approach content creation, data augmentation, and problem-solving across various industries. As these models continue to evolve, they raise important questions about the future of creative processes, the authenticity of digital content, and the ethical considerations surrounding AI-generated media. Understanding diffusion models is crucial for anyone interested in the cutting edge of AI technology. Whether you’re a researcher, a business leader, a policymaker, or simply curious about technological advancements, being aware of diffusion models provides insight into the rapidly evolving landscape of artificial intelligence and its potential impact on society.
In the following sections, we’ll delve deeper into how diffusion models work, their applications, challenges, and future prospects, providing you with a comprehensive understanding of this transformative technology.
How Diffusion Models Work
At their core, diffusion models operate by introducing noise to existing data and then learning to reverse this process. This involves two key phases:
- The Forward Process: Adding Noise
- Forward Process: The model starts with clear data (e.g., an image) and progressively adds Gaussian noise until it becomes indistinguishable from random noise.
Imagine you have a crystal-clear image of a cat. The forward process is like gradually sprinkling more and more sand onto this image until it becomes completely obscured. Here’s how it works:
- Starting Point: We begin with our clear, original data (like our cat image).
- Step-by-Step Noise Addition: The model adds small amounts of random noise to the data in multiple steps.
- Gaussian Noise: The type of noise added follows a Gaussian (or normal) distribution, chosen for its mathematical properties.
- Progressive Degradation: With each step, our cat image becomes less recognizable, more like a grainy, static-filled screen.
- Final State: After many steps, our once-clear cat image has transformed into pure noise, indistinguishable from random pixels.
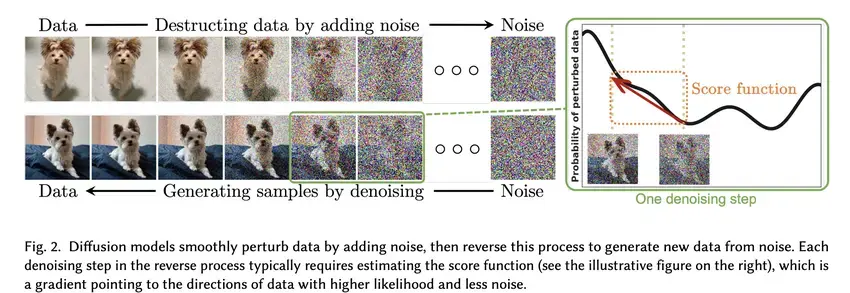
- The Reverse Process: The Art of Denoising
Now comes the magic. The reverse process is where the model learns to turn that noisy mess back into a coherent image. But here’s the kicker – it doesn’t necessarily recreate the original cat image. Instead, it generates a new, unique image that could plausibly have been the starting point for that noise. Here’s how:
- Starting Point: We begin with pure noise – that static-filled screen from the end of the forward process.
- Learned Reconstruction: The model has been trained to predict and remove small amounts of noise at each step.
- Iterative Refinement: Step by step, the model chips away at the noise, revealing more structure with each iteration.
- Emerging Clarity: As the process continues, recognizable features start to appear. Maybe we see an ear, then whiskers, then a full cat face.
- Final Output: The process results in a clear, coherent image – a brand new cat that never existed before but looks just as real as any photograph.
Applications in Various Fields
1. Image Generation:
Diffusion models have revolutionized the field of image generation, offering unprecedented quality and control. Here’s a deeper look:
Text-to-Image Generation:
Models like DALL-E, Midjourney, and Stable Diffusion have captured public imagination with their ability to create realistic or artistic images from text descriptions. For example, given a prompt like “a surrealist painting of a cat playing chess with an astronaut on the moon,” these models can generate a detailed, coherent image matching that description.How it works: The model interprets the text prompt, mapping it to features in its learned latent space. It then uses this as a guide during the denoising process to generate an image that matches the description.
Image Enhancement:
Diffusion models excel at improving image quality, including:
- Super-resolution: Upscaling low-resolution images while adding realistic details.
- Denoising: Removing noise and artifacts from images.
- Colorization: Adding color to black-and-white images.
Inpainting and Outpainting:
These models can fill in missing or corrupted parts of images (inpainting) or extend images beyond their original boundaries (outpainting). This is particularly useful in photo restoration or creative editing.
Medical Imaging:
In healthcare, diffusion models are making significant impacts:
- Improving MRI and CT scan quality, potentially reducing radiation exposure or scan times.
- Generating synthetic medical images for training AI systems, addressing data scarcity issues.
- Enhancing diagnostic accuracy by providing clearer, more detailed medical images.
2. Audio and Music Generation:
Diffusion models are pushing the boundaries of audio synthesis, offering new possibilities in music production and sound design.
Music Generation:
- These models can create original musical compositions in various styles and genres.
- They can extend or complete partial musical pieces, assisting composers in the creative process.
- Some models can generate music that matches a given mood, tempo, or even visual input.
Sound Effect Synthesis:
- Diffusion models can create realistic sound effects for film, gaming, and virtual reality applications.
- They can synthesize complex environmental sounds, like rain, crowds, or traffic, with high fidelity.
Voice Synthesis:
- These models are advancing text-to-speech technology, creating more natural and expressive voices.
- They can clone voices or create entirely new, artificial voices for various applications.
Audio Restoration:
- Similar to image restoration, diffusion models can clean up and enhance audio recordings, removing noise and improving clarity.
The potential here is vast, offering new tools for musicians, sound designers, and audio engineers to explore innovative soundscapes and push the boundaries of audio creation.
3. Text Generation:
While large language models like GPT have dominated headlines in text generation, diffusion models are making interesting contributions to this field as well.
Creative Writing Assistance:
- Diffusion models can help generate creative text, including poetry, short stories, or even elements of longer narratives.
- They can provide varied options for continuing a given text prompt, offering writers new ideas and directions.
Chatbots and Conversational AI:
- These models can generate more diverse and context-appropriate responses in chatbot applications.
- They offer potential improvements in maintaining conversation coherence over longer exchanges.
Content Creation:
- Diffusion models can assist in generating marketing copy, product descriptions, or other types of content.
- They can help create variations of existing text, useful for A/B testing in marketing or personalizing content.
Language Translation:
- Some researchers are exploring the use of diffusion models to improve machine translation, potentially offering more nuanced and context-aware translations.
The key advantage of diffusion models in text generation is their ability to produce diverse outputs from a single prompt, offering more varied and potentially more creative results compared to traditional language models.
4. Scientific Research:
Diffusion models are making significant contributions to various scientific fields, particularly in areas involving complex structures or processes.
Drug Discovery and Development:
- These models can generate and optimize molecular structures, potentially accelerating the drug discovery process.
- They can predict how drugs might interact with specific proteins or receptors, aiding in the design of more effective medications.
Protein Folding:
- Diffusion models are being used to predict protein structures, a crucial step in understanding biological processes and developing new treatments.
Materials Science:
- These models can simulate and predict the properties of new materials, assisting in the development of advanced materials for various applications.
Climate Modeling:
- Researchers are exploring the use of diffusion models to improve climate simulations, potentially enhancing our ability to predict and understand climate change.
Particle Physics:
- In high-energy physics, diffusion models are being used to simulate particle interactions, helping researchers analyze complex data from particle accelerators.
The application of diffusion models in scientific research is particularly exciting because it allows for the exploration of complex, high-dimensional spaces that are difficult or impossible to fully explore through traditional experimental methods. This can lead to new insights, accelerate discovery processes, and potentially revolutionize how we approach complex scientific problems.
In each of these fields, diffusion models offer a powerful tool for generating complex, realistic data. Their ability to learn and replicate intricate structures and patterns makes them versatile across a wide range of applications, from creative endeavors to cutting-edge scientific research. As the technology continues to advance, we can expect to see even more innovative applications emerge across various domains.
Challenges and Future Directions
- Computation Time:
Diffusion models operate by gradually transforming noise into structured data through a series of small steps. This iterative process, while effective, can be computationally expensive, especially for complex tasks or high-resolution outputs.
- The number of steps: Typically, diffusion models require hundreds or thousands of steps to generate high-quality results. Each step involves running the model, which adds up quickly.
- Hardware requirements: To mitigate the time issue, powerful GPUs or TPUs are often necessary, which can be costly and energy-intensive.
- Scalability concerns: As we aim to generate larger, more complex outputs (e.g., high-resolution images or long-form text), the computational demands grow significantly.
Research is ongoing to optimize these models and reduce the number of required steps without sacrificing quality. Techniques like diffusion distillation and improved sampling methods show promise in this area.
- Ethical Considerations:
The power of diffusion models to generate highly realistic content raises important ethical questions:
- Deepfakes: These models can potentially create very convincing fake images, videos, or audio, which could be used for misinformation or fraud.
- Copyright infringement: There are concerns about models trained on copyrighted material being used to generate derivative works without proper attribution or compensation.
- Bias and representation: Like all AI models, diffusion models can potentially amplify societal biases present in their training data.
- Privacy concerns: The ability to generate realistic personal data (e.g., faces, voices) raises questions about consent and data protection.
Addressing these issues requires a multifaceted approach involving technical solutions (e.g., watermarking generated content), policy development, and ongoing ethical discussions within the AI community and society at large.
- Controllability:
While diffusion models offer some degree of control through their step-by-step generation process, achieving precise and intuitive control remains a challenge:
- Attribute manipulation: Researchers are working on methods to allow users to specify and modify particular attributes of the generated content (e.g., changing the style of an image while preserving its content).
- Composition and layout: Controlling the overall structure and arrangement of elements in generated content (especially for images) can be difficult.
- Text-to-image alignment: Improving the models’ ability to accurately generate images based on detailed text descriptions is an active area of research.
- Temporal consistency: For video generation, maintaining coherence across frames while allowing for controlled changes is challenging.
Recent advances in this area include techniques like classifier-free guidance, which allows for better control over the generation process without requiring additional training. However, achieving the level of controllability seen in traditional digital creation tools remains an ongoing challenge.
Conclusion
Diffusion models represent a significant leap forward in the field of generative AI. By breaking down the complex task of data generation into a series of small, manageable steps, these models have opened up new possibilities in creating high-quality, diverse data across various domains. Their unique approach of gradually refining noise into structured information has led to remarkable advancements in image generation, audio synthesis, and even 3D modeling.
This step-by-step process not only produces outputs of exceptional quality but also allows for greater flexibility and control in the generation process. As research continues, we can expect to see even more impressive applications and refinements of this powerful technique, potentially transforming how we approach creative tasks and data synthesis in numerous fields.
Diffusion models represent a significant advancement in generative AI, tackling the task of data creation by gradually transforming random noise into meaningful outputs. This method has led to breakthroughs in fields like image generation, audio synthesis, and 3D modeling. Much like how GraphRAG has redefined retrieval-augmented generation by leveraging graph structures, diffusion models provide a powerful approach for generating high-quality, diverse data with greater control and flexibility.
For those familiar with Llama 3.2 and its revolutionizing of open-source multimodal AI, diffusion models similarly expand the possibilities for creative and technical applications. As these models continue to evolve and integrate with other AI techniques, their potential to shape industries like entertainment, research, and design will only grow, making diffusion models a key area to watch in the future of AI.