The 5 Best Image-Based Virtual Try-On AI Models: Pros, Cons, and Why They Matter
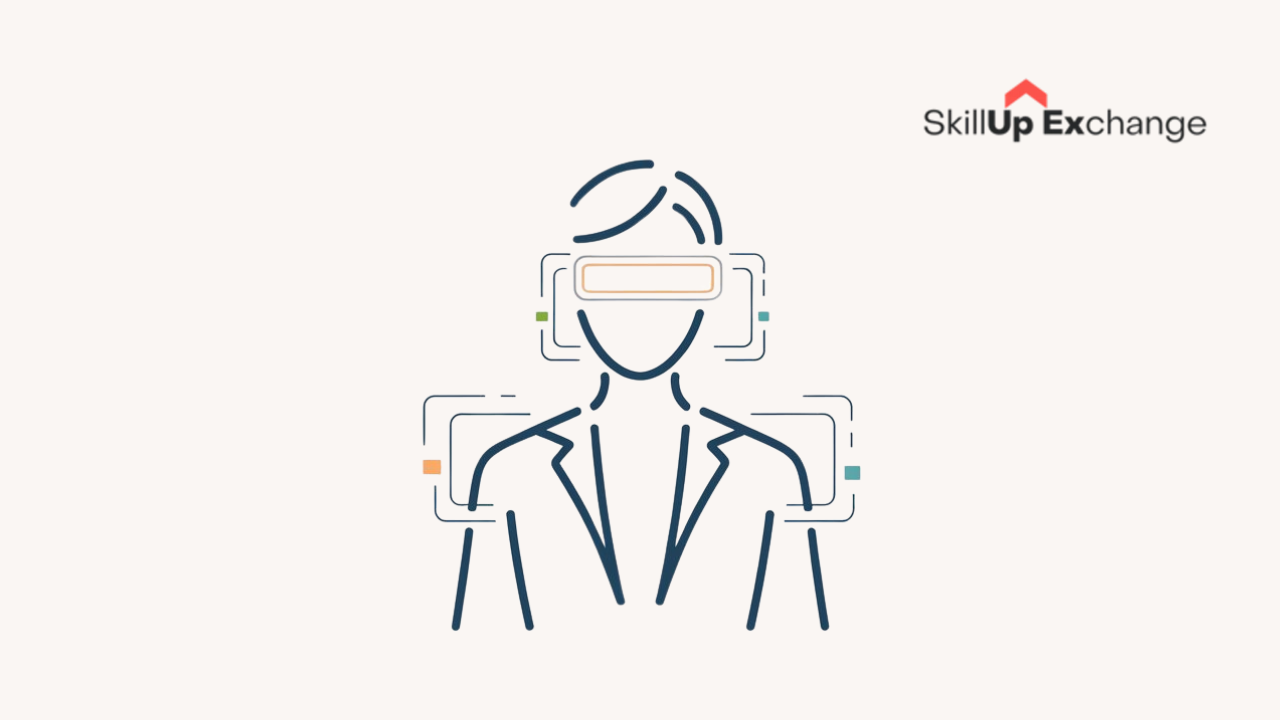
The 5 Best Image-Based Virtual Try-On AI Models: Pros, Cons, and Why They Matter
Image-based virtual try-on technology is transforming how we shop online, blending augmented reality, computer vision, and generative AI to let customers visualize products in real time. From reducing returns to boosting engagement, these tools are reshaping e-commerce. But with so many options, which models stand out? Below, we break down the five leading solutions, their strengths, weaknesses, and what makes them worth your attention.
Why Virtual Try-On AI Matters Now
Virtual try-on tools bridge the gap between online and in-store shopping by allowing customers to see how clothes, glasses, or makeup look on them digitally. Retailers using these systems report higher conversion rates and fewer returns, while brands like Warby Parker and Sephora have set new standards for immersive shopping. For businesses, the right AI model can mean the difference between a one-time buyer and a loyal customer.
The Top 5 Image-Based Virtual Try-On AI Models
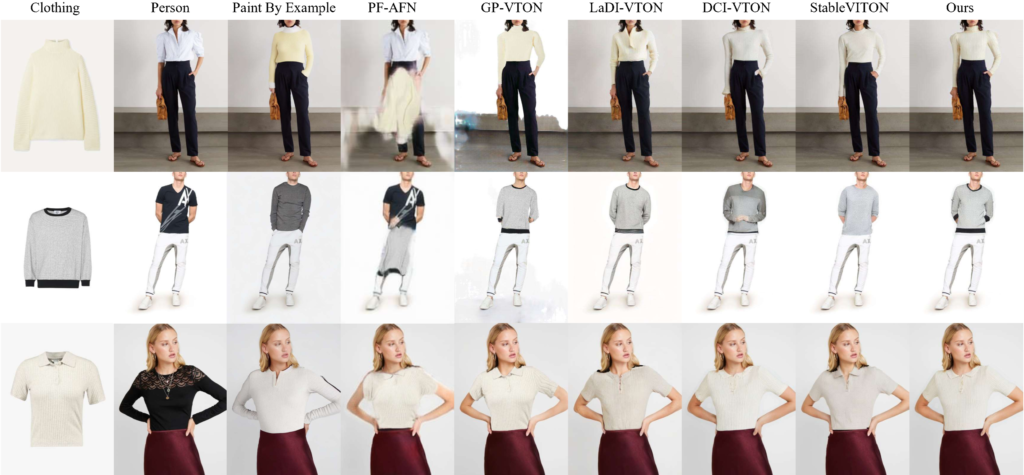
1. MV-VTON
Pros of MV-VTON
Generates realistic multi-view try-on results using both front and back clothing images, overcoming limitations of single-view approaches .
Employs diffusion models for superior image quality and detail compared to traditional methods .
Adapts clothing features to the person’s pose using view-aware selection and attention mechanisms, reducing alignment errors .
Cons of MV-VTON
Relies on having both front and back clothing images, which may not always be available .
Diffusion models require significant computational resources, increasing deployment costs .
Performance may suffer with very complex or unconventional poses .
May need fine-tuning for specific garment types or cultural attire outside its training data .
Why Choose MV-VTON?
Choose MV-VTON for high-fidelity, multi-angle virtual try-ons, particularly when accurate pose adaptation is crucial. Its advanced architecture excels in realistic rendering for diverse viewing angles.

2. CAT-DM
Pros of CAT-DM
- Enhanced Controllability: Combines diffusion models with ControlNet to improve garment pattern accuracy and alignment .
- Accelerated Sampling: Uses a pre-trained GAN to reduce sampling steps, enabling real-time virtual try-on while maintaining quality .
- High-Fidelity Results: Generates realistic images with detailed textures, outperforming both GAN-based and diffusion-based methods .
Cons of CAT-DM
- Complex Implementation: Requires integration of multiple models (ControlNet, GAN, diffusion), increasing technical complexity .
- Computational Costs: Despite acceleration, diffusion models still demand significant GPU resources for training .
- Dataset Dependency: Performance may vary on niche datasets (e.g., cultural attire) without fine-tuning .
Why Choose CAT-DM?
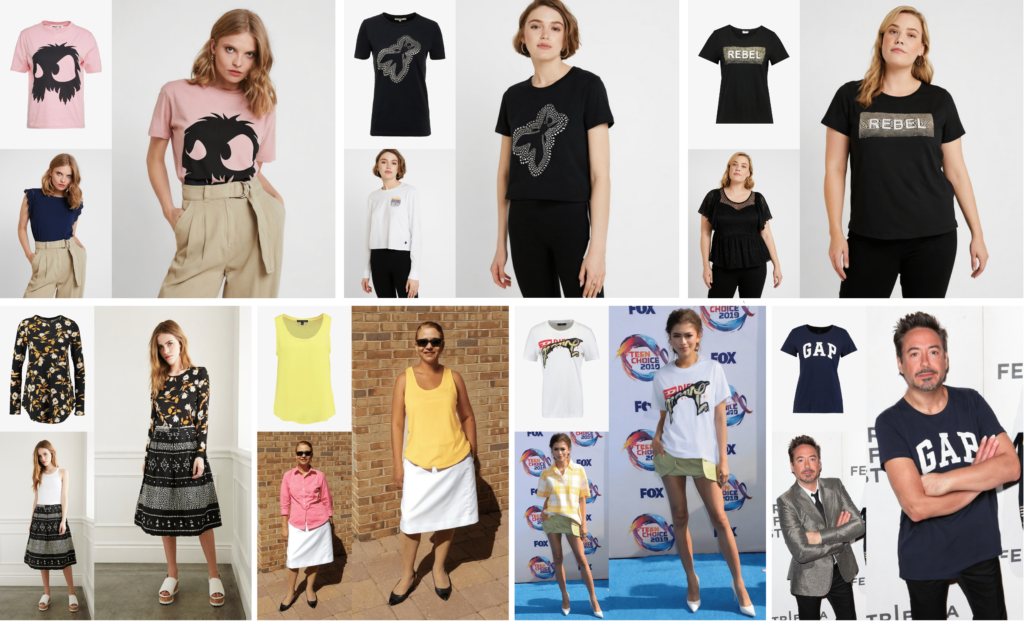
3. StableVITON
Pros of StableVITON
- High-Fidelity Results: Leverages pre-trained diffusion models to generate high-quality images with detailed clothing preservation .
- Semantic Correspondence: Uses zero cross-attention blocks to align clothing and human body features effectively, enhancing realism .
- Robust Generalization: Outperforms baselines in both single and cross-dataset evaluations, showing strong performance with arbitrary person images .
Cons of StableVITON
- Complex Implementation: Requires conditioning on multiple inputs (agnostic map, mask, dense pose), increasing implementation complexity .
- Computational Cost: Diffusion models are computationally intensive, potentially limiting real-time applications .
- Dataset Dependency: Performance may vary with different datasets, requiring careful fine-tuning for specific use cases .
Why Choose StableVITON?
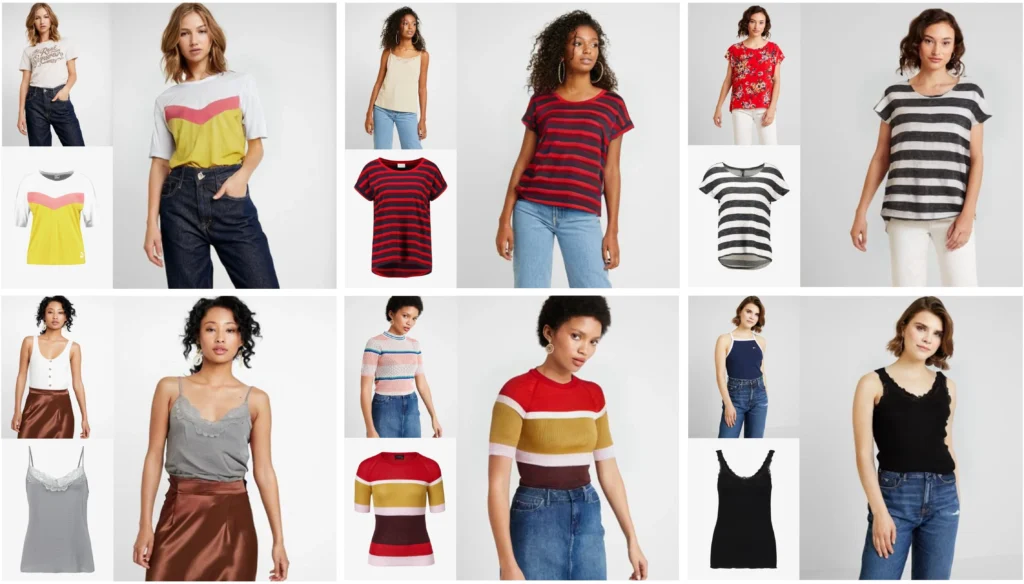
4. TPD
Pros of TPD
- High-Fidelity Texture Preservation: Utilizes diffusion models to maintain detailed garment textures and patterns, ensuring realistic virtual try-on results .
- Robust Generalization: Demonstrates strong performance across diverse datasets, including VITON-HD, with minimal artifacts .
- Efficient Training: Leverages pre-trained models (e.g., Paint-by-Example) for faster convergence and improved stability .
Cons of TPD
- Computational Demands: Diffusion models require significant GPU resources for training and inference, limiting accessibility for small-scale applications .
- Dataset Dependency: Performance may degrade on datasets with limited diversity or specific garment types (e.g., cultural attire) without fine-tuning .
- Implementation Complexity: Requires careful setup of data pipelines and model modifications (e.g., adjusting input channels), increasing technical barriers .
Why Choose TPD?

5. Any2AnyTryon
Pros of Any2AnyTryon
- Versatile and Mask-Free: Generates high-quality virtual try-on results without relying on masks or specific poses, offering flexibility and user-friendliness .
- Large-Scale Training Data: Utilizes the comprehensive LAION-Garment dataset for robust training across diverse garment types and tasks .
- Adaptive Position Embedding: Improves consistency and accuracy by aligning input images and generated outputs using dynamic position information .
Cons of Any2AnyTryon
- Complex Implementation: Requires handling variable input sizes and resolutions, increasing technical complexity .
- Computational Resources: Training and refining the model demands significant GPU memory and processing power .
- Network Issues: Public project resources (e.g., demo pages) may be temporarily unavailable or inaccessible due to network problems, as seen in the links provided .
Why Choose Any2AnyTryon?
Key Considerations When Choosing a Model
Factor | Priority | Why It Matters |
Realism | High | Poor rendering erodes trust; aim for natural shadows and fabric movement. |
Speed | Medium | Delays >1 second increase cart abandonment. |
Scalability | High | Ensure the model handles peak traffic without lag. |
Customization | Medium | Adapt to your product range (e.g., shoes vs. dresses). |